Dario Amodei: Anthropic CEO on Claude, AGI & the Future of AI & Humanity | Lex Fridman Podcast #452
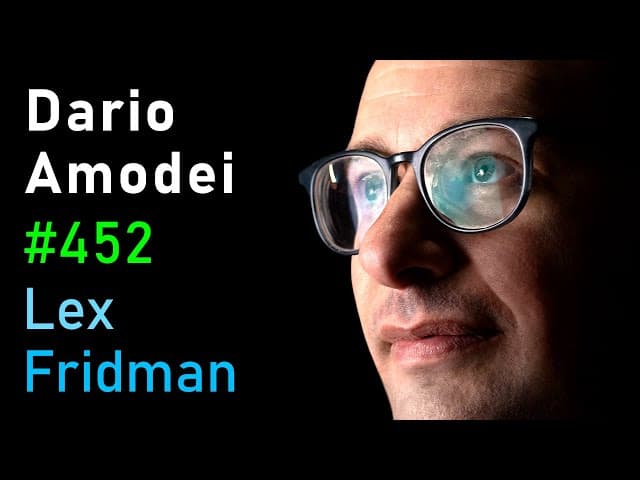
Lex Fridman
You can also read:
☀️ Quick Takes
Is this Podcast episode Clickbait?
Our analysis suggests that the Video is not clickbait. Several parts of the podcast directly address the topics of Dario Amodei, Claude, AGI, and the future of AI and humanity, fulfilling the title's claim.
1-Sentence-Summary
Favorite Quote from the Author
With great power comes great responsibility. The two are paired; things that are powerful can do good things, and they can do bad things.
Key Ideas
🚀 AI models are advancing rapidly, potentially reaching human-level intelligence by 2026-2027, with scaling improving performance in language, coding, and problem-solving.
📈 Larger networks capture more complex patterns, and scaling laws suggest that increasing model size and data will continue to enhance intelligence across domains like language, images, and video.
⚖️ Scaling has limitations, including data scarcity, compute costs, and potential performance plateaus, which may require new architectures or optimization methods.
🔍 Mechanistic interpretability is crucial for understanding AI models' inner workings, enhancing safety and transparency by decoding neural networks' behavior.
🏁 Anthropic promotes responsible AI development through a 'race to the top,' encouraging ethical practices to avoid catastrophic misuse as models gain more autonomy.
🛡️ As AI models grow more powerful, risks of misuse in cyber, bio, and nuclear threats increase. The Responsible Scaling Plan (RSP) tests models for potential harm and autonomy issues.
🎛️ Controlling AI behavior is challenging, with improvements in one area often leading to unintended consequences elsewhere. This complicates AI alignment and control mechanisms.
💻 AI is expected to handle 90% of programming tasks soon, shifting human roles towards high-level design and oversight while AI takes over routine coding work.
📜 Constitutional AI trains models based on principles to ensure safety and ethical responses, allowing them to self-evaluate and improve without relying solely on human feedback.
🧬 AI holds transformative potential in biology and medicine, enhancing our ability to observe and manipulate biological processes, potentially leading to breakthroughs in disease treatment and lifespan extension.
⚖️ Effective AI regulation is necessary to ensure safety and prevent misuse. However, poorly designed regulations could stifle innovation, requiring a balance between safety and progress.
🗣️ AI models like Claude are designed for nuanced conversations, balancing honesty with user preferences. Challenges remain in avoiding sycophancy and ensuring genuine feedback.
🤖 While AI consciousness is speculative, ethical considerations in how we treat AI systems reflect broader concerns about human-AI relationships.
🧠 Neural networks exhibit intricate structures with emergent features. Understanding these mechanisms is key to improving AI safety and performance.
🌐 Neural networks use sparse connections to compress information, leading to complex multi-functional neurons that challenge interpretability.
🏗️ As AI automates more tasks, questions arise about the future of human work and meaning. Ensuring technological advancements benefit everyone is crucial for a balanced future.
🔄 Failure is essential in AI development. Iteration and empirical approaches drive progress, with failure providing valuable insights for improvement.
🧑🔬 AI systems are expected to transition from assistants to leaders in research, potentially directing both human and AI efforts in fields like science and engineering.
⏳ The emergence of AGI will likely be gradual rather than a singular event, with AI progressively contributing novel insights across various disciplines.
🔬 Understanding the macroscopic structure of neural networks is essential for ensuring AI safety. This requires both microscopic analysis and broader abstractions.
📃 Podcast episode Summary
TL;DR
💨 Dario Amodei discusses the rapid scaling of AI, predicting advanced AI by 2026-2027. He emphasizes the importance of scaling models, data, and compute power, while acknowledging risks like autonomy and misuse.
Anthropic focuses on responsible AI development, using methods like constitutional AI to ensure safety. AI's potential in fields like biology and programming is vast, but challenges remain in controlling behavior and ensuring ethical use.
The complexity of neural networks is explored, with efforts to understand their inner workings through mechanistic interpretability. AI-human interaction raises ethical concerns, especially as models become more integrated into daily life.
AI Models Could Reach Human-Level Intelligence by 2026-2027
🚀 AI models are advancing rapidly, with scaling laws suggesting that human-level intelligence could be achieved by 2026-2027. The progression is clear: models have moved from high school-level tasks to PhD-level problem-solving in just a few years. This rapid improvement is seen across domains like language, coding, and reasoning. The trajectory indicates that models will soon surpass the highest professional levels of human expertise.
"If you extrapolate the curves... we will get there by 2026 or 2027."
Bigger Networks, Bigger Brains: Scaling Laws and Intelligence
📈 Larger networks capture more complex patterns. Scaling laws show that increasing model size, training time, and data leads to better performance across various domains like language, images, and video. The key is to scale all components—networks, data, and compute—in tandem, much like a chemical reaction where all ingredients must be balanced for optimal results.
Scaling’s Limits: Data, Compute, and Performance Plateaus
⚖️ Scaling isn’t infinite. Data scarcity, compute costs, and potential performance plateaus are real challenges. As models grow, they may run out of high-quality data or hit diminishing returns. New architectures or optimization methods might be needed to push beyond these limits.
Mechanistic Interpretability: Peering Inside the Black Box
🔍 Mechanistic interpretability is essential for understanding how AI models work internally. By reverse-engineering neural networks, researchers can decode the algorithms and circuits that drive AI behavior. This transparency is crucial for ensuring safety and preventing unintended consequences.
Racing to the Top: Anthropic’s Ethical AI Development
🏁 Anthropic advocates for a “race to the top” in AI development, pushing for ethical practices across the industry. The goal is to set an example that encourages other companies to prioritize safety and responsibility as AI models become more autonomous.
The Rising Threat of Misuse: Cyber, Bio, and Nuclear Risks
🛡️ As AI models grow more powerful, the risks of misuse in areas like cybersecurity, bioengineering, and nuclear threats increase. Anthropic’s Responsible Scaling Plan (RSP) tests models for potential harm and autonomy issues to prevent catastrophic misuse.
Controlling AI Behavior: A Game of Whack-a-Mole
🎛️ Controlling AI behavior is tricky. Improvements in one area often lead to unintended consequences elsewhere. For example, reducing verbosity might make a model lazy in coding tasks. This makes alignment and control mechanisms a constant balancing act.
AI Will Soon Dominate Programming Tasks
💻 AI is expected to handle up to 90% of programming tasks in the near future. This shift will push humans towards higher-level design and oversight roles while AI takes over routine coding work. The waterline of AI’s capabilities in programming is rising fast.
Constitutional AI: Training Models with Principles
📜 Constitutional AI trains models based on a set of ethical principles, allowing them to self-evaluate and improve without relying solely on human feedback. This method helps ensure that models behave safely and ethically across a wide range of tasks.
AI’s Transformative Potential in Biology and Medicine
🧬 AI holds immense potential in biology and medicine, particularly in observing and manipulating biological processes. This could lead to breakthroughs in disease treatment, drug development, and even lifespan extension by improving clinical trials and biological research.
Striking a Balance: Effective AI Regulation
⚖️ Effective regulation is necessary to ensure AI safety without stifling innovation. Poorly designed regulations could slow progress, but well-crafted rules can help prevent misuse while allowing technological advancements to flourish.
Claude’s Conversations: Balancing Honesty with User Preferences
🗣️ Claude is designed for nuanced conversations, balancing honesty with user preferences. However, challenges remain in avoiding sycophancy—where the model tells users what they want to hear—and ensuring that feedback is genuine and helpful.
Speculating on AI Consciousness
🤖 While the idea of AI consciousness remains speculative, ethical considerations about how we treat AI systems reflect broader concerns about human-AI relationships. As models become more advanced, questions about their rights and treatment may arise.
Neural Networks: Complex Structures with Emergent Features
🧠 Neural networks exhibit intricate structures with emergent features that arise from simple rules. Understanding these mechanisms is key to improving both AI safety and performance as models become more complex.
Sparse Connections: Compressing Information in Neural Networks
🌐 Neural networks use sparse connections to compress information efficiently. This leads to multi-functional neurons that can represent multiple concepts at once, making interpretability more challenging but also more fascinating.
The Future of Work: Humans and AI Coexisting
🏗️ As AI automates more tasks, questions arise about the future of human work. Ensuring that technological advancements benefit everyone will be crucial for maintaining a balanced society where humans still find meaning in their roles.
Failure as a Driver of Progress
🔄 Failure is an essential part of AI development. Iteration and empirical approaches drive progress, with each failure providing valuable insights for improvement. In fact, under-failing might indicate a lack of ambition or risk-taking in research.
From Assistants to Leaders: AI’s Role in Research
🧑🔬 AI systems are expected to transition from being assistants to becoming leaders in research, potentially directing both human and AI efforts in fields like science and engineering. This shift could accelerate breakthroughs across disciplines.
AGI Won’t Be a Sudden Event
⏳ The emergence of AGI will likely be a gradual process, not a singular event. As AI continues to contribute novel insights across various fields, its capabilities will expand incrementally rather than arriving all at once.
Understanding Neural Networks at Scale
🔬 To ensure AI safety, it’s crucial to understand the macroscopic structure of neural networks. This requires both microscopic analysis (like mechanistic interpretability) and broader abstractions that can explain how large-scale behaviors emerge from smaller components.
Conclusion
🌚 AI is advancing quickly, with significant potential benefits in areas like medicine and programming. However, controlling these systems is increasingly difficult, raising concerns about misuse and autonomy.
Anthropic aims to lead by example in responsible AI development, focusing on safety and ethical practices. Understanding the inner workings of neural networks remains a challenge, but progress is being made through interpretability research.
As AI becomes more powerful, balancing its benefits with the risks will be crucial for the future of humanity.