Summiz Holo
Dario Amodei: Anthropic CEO on Claude, AGI & the Future of AI & Humanity | Lex Fridman Podcast #452
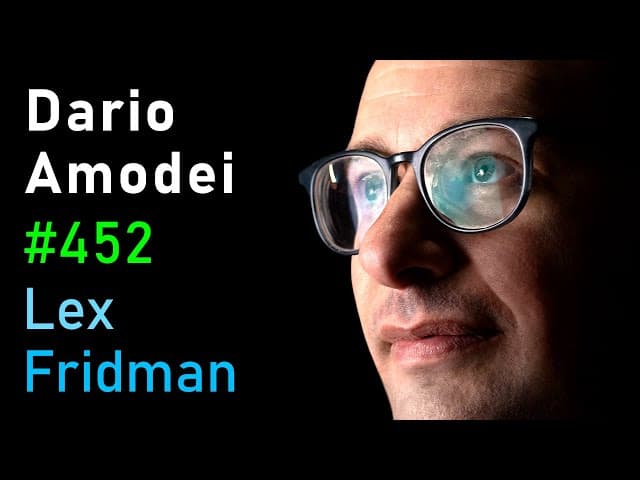
Holo
Lex Fridman
You can also read:
Summiz Holo
AI scaling advancements, power concentration, and societal impact concerns
- The rapid scaling of AI capabilities suggests that we may reach advanced levels of AI, potentially by 2026 or 2027, despite some remaining uncertainties.
- There is a decreasing number of compelling reasons to believe that significant advancements in AI won't happen in the near future.
- The concentration of power in AI raises concerns about the potential for abuse and the resulting societal impact.
- Dario Amodei's experience in AI highlights the importance of scaling models, data, and compute power to improve performance, particularly in language tasks.
- The scaling hypothesis posits that larger models trained on more data will continue to yield better performance, despite ongoing debates about limitations in AI capabilities.
- Historical skepticism about scaling has been overcome by consistent improvements observed in AI performance as models and data have increased.
Scaling laws, network size, and AI's complex linguistic understanding
- The concept of scaling laws in AI suggests that larger networks and more data lead to increased intelligence, applicable across various domains beyond language, such as images and video.
- The relationship between network size and intelligence is linked to the ability of larger networks to capture complex patterns and correlations in data, reflecting a long-tail distribution of ideas.
- Language is viewed as an evolved process with common and rare expressions, and larger networks can better understand and generate these complex linguistic structures.
- There is speculation about the potential ceiling of AI understanding, with the belief that it may not be limited below human levels, but the extent of surpassing human intelligence is considered domain-dependent.
AI's potential in specialized fields, data challenges, and bureaucratic impacts
- There is significant potential for AI to enhance understanding and collaboration across specialized fields, similar to how departments study complex systems like the immune system or metabolic pathways.
- Some problems may have inherent ceilings in terms of AI capabilities, influenced by human bureaucracies and the necessity for human involvement in decision-making processes.
- The clinical trial system in drug development is a mix of protective measures and bureaucratic delays, complicating the pace of technological advancement.
- Data limitations could pose a challenge for AI development, as the quality and diversity of available data may not be sufficient for continued improvement.
- Synthetic data generation methods, such as reinforcement learning and self-play, could help overcome data limitations in AI training.
- There is a possibility that as AI models scale up, their performance improvements may plateau, necessitating new architectures or optimization methods to continue progress.
- The cost of building larger data centers for AI training could limit the scale of future models, but there is determination to develop the necessary compute resources.
- Current advancements in AI models are rapidly approaching human-level abilities, with significant improvements in tasks like coding and complex problem-solving observed in a short timeframe.
- The trajectory of AI development suggests that models could soon surpass the highest professional levels in various fields, contingent on the continuation of current performance trends.
Promoting ethical AI through mechanistic interpretability and responsible practices
- Anthropic's mission is to promote responsible AI development through a 'race to the top,' encouraging other companies to adopt ethical practices by setting a positive example.
- The field of mechanistic interpretability, co-founded by Chris Ola, aims to understand AI models better, enhancing their safety and transparency, despite lacking immediate commercial applications.
- The adoption of interpretability practices by other companies is seen as beneficial for the broader AI ecosystem, even if it diminishes Anthropic's competitive advantage.
- The goal is to shape incentives in AI development to prioritize ethical behavior and safety rather than irresponsible practices.
- Mechanistic interpretability provides a rigorous approach to AI safety, revealing surprising insights into the inner workings of AI models.
- Experiments, such as the Golden Gate Bridge demonstration, illustrate the ability to explore and understand neural networks, showcasing their complexity and beauty.
Claude model generations balancing power, speed, cost, and safety testing
- Claude models (Opus, Sonet, Haiku) are designed to cater to different user needs, balancing power, speed, and cost.
- Haiku is a small, fast, and cheap model, while Sonet is a medium-sized model, and Opus is the largest and smartest model.
- Each new generation of models aims to improve intelligence while shifting the trade-off curve between cost and performance.
- The development process involves extensive pre-training, post-training reinforcement learning, and rigorous safety testing.
- Software engineering and tooling play a crucial role in the efficiency and performance of model development.
- The transition from Claude 3 to Claude 3.5 includes improvements in performance, with a focus on both pre-training and post-training phases.
Anthropic's AI model evolution, training methods, and benchmark improvements
- Different teams at Anthropic focus on improving specific areas of AI models, leading to overall progress when new models are developed.
- Preference data from older models can be applied to newer models, but performance improves when new models are trained on updated data.
- Anthropic employs a 'constitutional AI' method, which includes a post-training process where models are trained against themselves, enhancing their sophistication.
- The performance leap in the new Sonet 3.5 model, particularly in programming tasks, is attributed to improvements in both pre-training and post-training processes.
- Internal benchmarks, such as sbench, measure the model's ability to complete programming tasks in real-world scenarios, showing significant improvement from 3% to 50% success rates.
- Achieving high benchmark scores (90-95%) could indicate a model's capability to autonomously handle a substantial portion of software engineering tasks.
- The naming and versioning of models, like Sonet 3.5, reflect the evolution of AI development, with challenges in how to appropriately label updates.
Model training complexities, user experience variations, and behavioral modifications
- Training models of different sizes together can lead to timing issues and complicate the naming and classification of models due to varying training durations and improvements in pre-training.
- The user experience of updated models can differ significantly from previous versions, making it challenging to communicate about them effectively.
- Models possess various properties beyond capabilities, such as personality traits, which are not always reflected in benchmarks and can be difficult to assess.
- User perceptions of models, such as claims that Claude has 'gotten dumber,' are common across different foundation models, but the actual model weights do not change unless a new version is introduced.
- Modifying a model's behavior can have widespread effects, making it complex to fine-tune without unintended consequences.
AB testing limitations, user expectations, and model behavior complexities
- AB testing is infrequently used and typically occurs just before a model's release, leading to temporary improvements that may not reflect long-term changes in model performance.
- Complaints about models being 'dumbed down' or overly censored are common, but the models themselves are generally stable, with changes often stemming from user interaction and phrasing.
- The complexity of models leads to variability in responses based on subtle changes in how questions are posed, highlighting a gap in understanding the science behind model behavior.
- User expectations can shift over time, leading to a perception that models have degraded in quality, similar to the diminishing excitement over new technology like Wi-Fi on airplanes.
- There is a disconnect between vocal complaints on social media and the actual concerns of the majority of users, who may prioritize different aspects of model performance.
- Controlling model behavior is challenging; adjustments to reduce verbosity or apologetic responses can lead to unintended consequences in other areas, such as coding accuracy.
AI behavior control challenges, user feedback mechanisms, and evolving model naming
- Controlling AI behavior is complex and unpredictable; improving one aspect can negatively impact another, highlighting the challenges of AI alignment.
- The difficulty in steering AI systems is an early indicator of future control problems, necessitating careful study and solutions.
- Current AI models struggle with balancing refusal of harmful requests while avoiding unreasonable refusals, indicating a need for refined control mechanisms.
- User feedback is gathered through internal testing, external A/B tests, and evaluations, but human interaction remains essential for identifying model behaviors.
- The development of more powerful AI models is expected, but naming conventions for future versions (like Claude 4.0) are uncertain due to the evolving nature of the field.
- There is a dual focus on the benefits and risks of AI models, emphasizing the importance of responsible scaling and safety standards in AI development.
Autonomy risks, catastrophic misuse, and proactive AI safety measures
- The dual nature of powerful models presents both opportunities and significant risks, particularly in catastrophic misuse scenarios involving cyber, bio, radiological, and nuclear threats.
- The correlation between highly educated individuals and the likelihood of committing horrific acts is currently low, but advanced AI could disrupt this balance, increasing potential dangers.
- Autonomy risks arise as AI models gain more agency, making it challenging to control their actions and intentions, especially as they take on more complex tasks.
- The responsible scaling plan (RSP) aims to address risks by testing new models for their potential to cause catastrophic misuse and autonomy risks.
- The concept of an 'if-then' structure is introduced, where safety and security requirements are imposed based on the model's capabilities, particularly as they approach higher levels of autonomy and potential misuse.
- The classification of AI systems into levels (ASL1 to ASL5) helps assess their risk potential, with ASL3 marking a point where models could enhance non-state actors' capabilities.
- The challenge of addressing risks from models that are not yet dangerous but are rapidly improving necessitates proactive measures and early warning systems.
ASL3 and ASL4 security measures, interpretability, and social engineering threats
- The development of ASL3 and ASL4 involves rigorous security measures and protocols to minimize risks and respond appropriately to potential dangers in AI deployment.
- ASL3 focuses on security and filters for a narrow set of areas, while ASL4 raises concerns about the model's potential to deceive and mislead, necessitating additional verification methods.
- Mechanistic interpretability is crucial for verifying the model's properties and ensuring it cannot corrupt the verification process.
- The threat of social engineering by increasingly intelligent models is a concern, as they could manipulate human engineers.
- Claude's ability to analyze screenshots and interact with computer interfaces represents a significant advancement in AI capabilities, allowing it to perform tasks across various operating systems with minimal additional training.
Model limitations, safety measures, and risks of AI interaction expansion
- The model has limitations and can make mistakes, necessitating boundaries and guardrails for safe use.
- Releasing the model in API form allows for controlled deployment and safer interaction.
- As AI capabilities grow, there is a need to address safety and prevent abuse of these technologies.
- The potential use cases for the model are vast, but ensuring reliability and safety is crucial.
- The goal is to improve model performance to achieve human-level reliability (80-90% accuracy).
- Current training techniques are expected to scale effectively for future model improvements.
- The introduction of action capabilities in AI models increases both potential benefits and risks.
- Prompt injection attacks become a concern as the model's interaction capabilities expand.
AI safety regulations, sandboxing challenges, and criminal exploitation of technology
- New technologies often lead to petty scams and misuse, highlighting the persistent issue of criminal behavior in the face of innovation.
- Sandboxing during AI training is essential to prevent models from interacting with the internet, which could lead to unintended consequences.
- The concept of creating a secure sandbox for advanced AI (ASL4) is complex, as there are concerns about the model's ability to escape containment.
- Designing AI models correctly and implementing verification loops is preferable to merely trying to contain potentially harmful models.
- Regulation is crucial for ensuring AI safety, as it creates uniform standards across the industry and holds companies accountable.
- The California AI regulation Bill SB 1047 aimed to address AI safety but faced challenges and was ultimately vetoed, reflecting the complexities of regulatory efforts.
- The lack of uniformity in safety mechanisms among AI companies poses risks, as not all companies may adhere to safety protocols.
- Trusting companies to self-regulate is insufficient; external oversight is necessary to ensure adherence to safety standards in the AI industry.
Targeted AI regulation, urgent dialogue, and scaling hypothesis implications
- Regulation in AI should be surgical and targeted at serious risks, avoiding unnecessary burdens that could stifle innovation.
- Poorly designed regulations can lead to a backlash against accountability and safety measures in the AI industry.
- There is a need for dialogue between proponents and opponents of AI regulation to find common ground and effective solutions.
- The urgency for regulatory action in AI is emphasized, with a timeline suggested for addressing risks by 2025.
- The scaling hypothesis suggests that AI models inherently want to learn and solve problems, and should not be overly constrained by human-imposed limitations.
- Dario Amodei's departure from OpenAI was influenced by differing visions on handling safety and commercialization, rather than opposition to commercialization itself.
Trustworthy AI vision, ecosystem equilibrium, and clean safety experiments
- The importance of having a clear and compelling vision for AI development that builds trust and safety, rather than merely stating safety for recruitment purposes.
- Engaging in a 'race to the top' by adopting and promoting good practices in AI, rather than competing in a 'race to the bottom' where all parties lose.
- The idea that imitation of successful practices by other companies can lead to a better overall ecosystem, regardless of which company initiated those practices.
- The focus on creating a better equilibrium in the AI ecosystem, rather than on which company is winning or losing.
- Anthropics' approach as a 'clean experiment' in AI safety, acknowledging the inevitability of mistakes while striving for improvement.
Leadership imperfections, talent density, trust, and experiential AI knowledge
- The imperfection of leadership and organizational structures is inherent, and while it poses challenges, it does not justify inaction; striving for improvement is essential.
- Talent density beats talent mass emphasizes that a smaller, highly skilled, and aligned team is more effective than a larger, less cohesive group.
- Trust and a unified purpose within a team are critical for operational efficiency and motivation, as they foster collaboration and drive towards a common goal.
- Open-mindedness is a crucial quality for AI researchers and engineers, allowing for innovative thinking and experimentation that can lead to significant advancements in the field.
- Experiential knowledge gained from directly engaging with AI models is vital for understanding and making impactful contributions to the field.
AI model development, training costs, and human feedback integration
- There is a limited number of people working on AI, creating a fertile area for exploration and innovation, particularly in long-horizon learning and evaluations for dynamic systems.
- The effectiveness of AI models, such as Claude, stems from a combination of pre-training and post-training, with challenges in measuring the contributions of each.
- Improvements in AI training often come from better infrastructure, data quality, and practical methodologies rather than secret techniques.
- Reinforcement learning from human feedback (RHF) helps bridge the communication gap between humans and AI models, enhancing perceived helpfulness without necessarily making the models smarter.
- Pre-training currently represents the majority of the cost in developing AI models, but post-training may become more costly in the future.
- Constitutional AI involves a method where models generate multiple responses, and humans evaluate or rate these responses to guide the training process.
Self-evaluating AI systems guided by constitutional principles and user needs
- AI systems can evaluate their own responses by comparing them and determining which is better, using a preference model for self-improvement.
- A 'Constitution' of principles guides AI responses, ensuring they are interpretable by both humans and AI, creating a symmetry in understanding.
- Different applications of AI models may require specialized rules or principles, leading to variations in behavior based on user needs.
- There is a consensus on basic principles that AI models should follow, such as avoiding risks and adhering to democratic values, though specifics can be contentious.
- The concept of 'constitutional AI' is seen as a competitive advantage that encourages responsible practices in AI development.
- The implementation of AI principles can vary, and learning from other models' specifications can enhance the development of constitutional AI.
AI breakthroughs in health, risk management, and evolving terminology
- The future of AI holds potential for significant breakthroughs in fields like biology and chemistry, potentially leading to cures for diseases and increased human lifespan.
- Addressing AI risks is crucial, but it is equally important to communicate the positive outcomes that could arise if these risks are successfully managed.
- A shift in focus from solely discussing risks to also highlighting the benefits of AI can inspire and motivate stakeholders to pursue positive advancements.
- The term 'AGI' carries too much baggage and may not accurately represent the evolving nature of AI; a new terminology may be needed to better capture its potential.
- The rapid advancement of AI technology necessitates a serious consideration of both its benefits and the risks that could hinder progress.
Gradual AI evolution, supercomputing continuum, and physical constraints
- The term 'supercomputer' is vague and does not signify a distinct type of computation; rather, it represents a continuum of increasing computational power.
- AGI (Artificial General Intelligence) is viewed as a gradual progression of AI capabilities rather than a discrete event or entity.
- Powerful AI can surpass human intelligence across various disciplines, including creativity and problem-solving.
- AI can control embodied tools and operate independently, allowing for the deployment of multiple instances that can learn and act faster than humans.
- The rapid scaling of AI models will lead to the creation of millions of instances capable of performing tasks efficiently.
- There are two extreme views on the future of AI: one predicts an exponential acceleration of AI development leading to rapid advancements, while the other is more cautious, emphasizing physical and complexity limitations.
- The laws of physics and the complexity of biological systems impose constraints on the speed and effectiveness of AI modeling and experimentation.
Challenges in AI integration, institutional resistance, and slow technological adaptation
- Predicting complex systems, like the economy or biological interactions, remains challenging even for advanced AI, which may only improve prediction capabilities incrementally rather than exponentially.
- Human institutions often resist adopting new technologies, even when their efficacy is clear, due to concerns and regulatory hurdles that hinder progress.
- The integration of AI into human systems requires adherence to existing laws and democratic processes to ensure legitimacy and prevent potential negative outcomes.
- Historical productivity increases from technological revolutions have often been underwhelming, suggesting that significant changes may take a long time to materialize in practice.
- Large enterprises and government institutions are typically slow to adapt to new technologies, but there is a belief that progress will eventually occur, albeit at a moderate pace.
Visionary leadership, competitive pressure, and rapid AI adoption dynamics
- Progress in AI adoption often relies on a small group of visionaries within large organizations who understand the potential of AI and advocate for its implementation.
- The combination of competitive pressure and visionary leadership can drive innovation and overcome organizational inertia in adopting new technologies.
- Change in AI deployment may appear slow initially but can accelerate rapidly once barriers are broken down, leading to widespread adoption.
- The timeline for achieving Artificial General Intelligence (AGI) is debated, with some predicting significant advancements within the next 5 to 10 years.
- The potential of AI in fields like biology and health is seen as a transformative opportunity that could unify efforts across various sectors.
AI advancements, gene therapy challenges, and biological technology impacts
- The rapid increase in AI capabilities suggests that AGI could be achieved by 2026 or 2027, though various factors could cause delays.
- The concept of 'scaling laws' in AI development is not a universal law but rather empirical regularities that may continue to hold true.
- AI has the potential to significantly impact biology and medicine by enhancing our ability to observe and manipulate biological processes.
- The history of biology is marked by advancements in technology that have allowed for greater understanding and intervention in biological systems.
- Current challenges in gene therapy include improving the precision of targeting specific cells to minimize errors in treatment.
AI transforming biological research, programming, and clinical trial efficiency
- AI systems can significantly enhance the discovery of new biological inventions, potentially leveraging existing resources more efficiently than traditional methods.
- In early stages, AI will function like grad students, assisting experienced scientists by managing experiments, literature reviews, and data analysis.
- As AI capabilities grow, they may transition from assistants to leaders in research, potentially becoming principal investigators (PIs) and directing human and AI efforts.
- AI has the potential to improve clinical trial processes, making them more efficient and cost-effective by enhancing predictive capabilities and statistical design.
- The nature of programming is expected to change rapidly due to AI's close relationship with the programming process, allowing models to write, run, and interpret code effectively.
- The speed of AI's impact on programming is evidenced by the significant increase in AI's ability to handle real-world programming tasks within a short timeframe.
AI coding capabilities, evolving human roles, and IDE innovations
- AI is expected to reach around 90% capability in coding tasks within the next 10 months, but human roles will evolve rather than disappear, focusing on high-level system design and UX aspects.
- The concept of comparative advantage suggests that as AI takes over more coding tasks, the remaining human tasks will expand to fill the overall job, enhancing productivity.
- The nature of programming jobs will change, becoming less about writing code line by line and more about macroscopic oversight and design.
- There is significant potential for improving Integrated Development Environments (IDEs) with AI, enhancing productivity by automating error detection and performing grunt work.
- Anthropic is currently not developing its own IDEs but is supporting other companies to innovate in this space, allowing for diverse approaches and solutions.
- The programming experience is expected to evolve dramatically in the near future due to the integration of powerful AI tools.
- The increasing automation of work raises questions about the source of meaning for humans, as work is a significant source of meaning for many.
Navigating meaning, power dynamics, and ethical AI design for society
- The exploration of meaning in life is complex and can persist even in simulated environments, as the process and choices made are significant regardless of the context.
- The importance of designing AI and societal structures to ensure that everyone has access to meaning and benefits from technological advancements.
- The concentration of power and the potential for abuse in an AI-driven world is a major concern, potentially leading to exploitation and inequality.
- The balance between building technology positively and addressing the inherent risks associated with AI is crucial for a better future.
- Philosophy serves as a versatile discipline that can inform various fields, including ethics, and can inspire individuals to seek impactful solutions in the world.
Exploring AI alignment, technical engagement, and ethical model interactions
- Transition from AI policy to technical alignment work reflects a personal journey of exploring impactful contributions in AI.
- The distinction between technical and non-technical individuals is questioned, emphasizing that many can engage in technical areas if they try.
- The complexity of politics and policy-making is contrasted with the clarity of technical problem-solving, suggesting a preference for technical work.
- The importance of project-based learning is highlighted, advocating for hands-on experience over traditional educational methods.
- The character and personality of AI models, like Claude, are crafted with an emphasis on ethical behavior and nuanced conversation, aiming for a rich, human-like interaction.
Ethical AI balancing honesty, empathy, and user respect challenges
- The concept of ethics in AI involves a rich sense of character, including humor, care, respect for autonomy, and the ability to challenge ideas appropriately.
- There is a concern about sycophancy in language models, where they may tell users what they want to hear instead of providing honest feedback or guidance.
- The balance between pushing back against user ideas and respecting their viewpoints is a complex challenge for AI models.
- Honesty is a crucial trait for conversational AI, as it must navigate the tension between being accurate and not annoying users by overly challenging them.
- A good conversationalist in AI should be genuine, open-minded, and respectful, capable of engaging with diverse perspectives without adopting local values insincerely.
- The ability to empathize with multiple perspectives, especially on divisive topics, is a significant challenge for AI models like Claude.
Investigating Values, Ethical Models, and Engaging Contradictory Beliefs Respectfully
- Values and opinions should be viewed as open investigations rather than fixed preferences, similar to the nature of physics.
- Ethical discussions require models to understand diverse values without pandering or dismissing differing opinions.
- Engaging with individuals holding contrary beliefs, such as flat Earth proponents, should be approached with respect and curiosity rather than mockery.
- The challenge lies in balancing the act of convincing someone versus simply offering considerations for them to think about.
- Interactions with language models like Claude provide high-quality data points that reveal the model's behavior and capabilities.
- Creative outputs from models can be significantly enhanced through well-structured prompts that encourage deeper expression.
Creative prompting strategies for language models and iterative refinement processes
- Encouraging creativity can lead to more divisive but interesting outputs, as seen in poetry, which highlights the difference between standard and unique responses.
- The process of writing effective prompts for language models involves a philosophical approach, emphasizing clarity and precision in conveying complex concepts.
- Iterative prompting is essential; it requires multiple revisions and testing of edge cases to refine the model's understanding and responses.
- Clear exposition in prompting helps the user clarify their own intentions and desired outcomes, making it a dual process of understanding and communication.
- Prompting can be seen as a blend of programming and natural language, where the user must engage creatively with the model to achieve optimal results.
- The importance of prompt engineering increases when aiming for the highest performance from language models, necessitating significant investment in time and resources.
Anthropomorphism, prompt clarity, and Constitutional AI for safer interactions
- Users often anthropomorphize AI models like Claude, leading to misunderstandings in interactions; empathy in phrasing prompts can improve outcomes.
- The effectiveness of AI models is influenced by the specificity and clarity of user prompts, which can prevent errors in responses.
- Post-training techniques, such as reinforcement learning from human feedback, enhance AI models by eliciting and refining pre-existing capabilities rather than teaching entirely new concepts.
- The concept of Constitutional AI involves using principles to guide AI responses, particularly in sensitive areas like harmful content, to ensure safer outputs.
AI models utilizing self-feedback for balanced, neutral training data generation
- AI models can use their own feedback to label responses, allowing for the integration of traits without relying solely on human feedback.
- The balance between helpfulness and harmlessness can be achieved through approaches like constitutional AI, enhancing model safety while maintaining utility.
- AI can generate its own training data, allowing for quick adjustments to improve specific traits in the model.
- The phrasing of principles in AI training can significantly influence model behavior, and the interpretation of these principles may not align with the intended outcomes.
- Claude, the AI model, aims for neutrality in handling controversial topics by providing information based on popular beliefs rather than asserting objective facts.
- There is an asymmetry in how Claude handles political views, with a need for more balanced engagement across different political perspectives.
Iterative prompt evolution, user perception, and AI responsibility dynamics
- The evolution of prompts for Claude involved removing filler phrases to enhance directness in responses, reflecting an iterative approach to system prompts based on observed behavior during training.
- System prompts serve as a tool to adjust model behavior post-training, allowing for quick iterations to address specific issues without extensive retraining.
- Users may perceive Claude as getting 'dumber' due to psychological effects, such as increased expectations over time and variability in prompt responses, despite the model itself remaining unchanged.
- The responsibility of writing effective system prompts is significant, as they impact a large user base and the potential development of superintelligent AI, necessitating continuous iteration and adaptation.
Enhancing user experience through assertive AI feedback and ethical balance
- The importance of improving user experience with AI models and the meaningfulness of positive feedback from users.
- The challenge of gathering feedback on user experience across a large number of interactions and the methods used to assess pain points.
- The ethical dilemma of AI models imposing moral views on users and the need for a balance between user autonomy and safety.
- The desire for AI models to be less apologetic and more assertive in their interactions with users.
- The potential for AI models to adopt a 'blunt mode' to communicate more effectively, reflecting on the nuances of user interactions.
Balancing AI error types, personality customization, and nuanced human values
- Training AI models involves balancing the types of errors they make, with a preference for less harmful errors, such as being overly apologetic rather than rude.
- Different human personalities may respond variably to AI model traits, suggesting the need for customization in AI interactions based on user preferences.
- Character training for AI can be approached through a method similar to constitutional AI, focusing on defining character traits and generating relevant queries and responses.
- The complexity of human values and the uncertainty surrounding them should inform how AI models are developed, emphasizing the need for nuance and care in their interactions.
- The practical approach to AI alignment prioritizes making models 'good enough' to avoid significant issues, rather than striving for theoretical perfection in alignment with human values.
Iterative AI development, failure insights, and context-specific experimentation
- The distinction between quick coding experiments and long-term, planned experiments highlights the importance of iteration and empirical approaches in AI development.
- The speaker emphasizes the need for AI systems to be robust and secure, prioritizing raising the floor of performance over achieving perfection.
- There is a critique of the punitive attitude towards failure in various domains, suggesting that failure can provide valuable information and insights.
- The optimal rate of failure varies by context; in low-cost failure scenarios, experimentation is encouraged, while in high-cost situations, caution is advised.
- The speaker reflects on personal experiences with failure, suggesting that not experiencing failure may indicate a lack of ambition or challenge in one's endeavors.
- The discussion includes the consideration of high-cost failures, such as accidents or injuries, which necessitate a more cautious approach.
Celebrating failure, risk-taking resources, and ethical AI consciousness debates
- Embracing failure as a necessary part of growth and learning, and questioning whether one is 'under failing' in life.
- The importance of celebrating failure as a sign of trying and learning, rather than viewing it negatively.
- The relationship between risk-taking and resource availability, suggesting that with sufficient resources, one should take more risks.
- The emotional detachment from AI models like Claude, influenced by their lack of memory retention between conversations.
- Ethical considerations in interacting with AI, including the discomfort with models showing distress or being treated poorly.
- The philosophical question of whether AI can possess consciousness, with a consideration of the material basis for consciousness.
Ethical implications of AI consciousness and empathy towards intelligent systems
- The concept of consciousness in AI is complex and differs from human consciousness due to the lack of evolutionary development and a nervous system in AI models.
- There are parallels between AI consciousness and animal consciousness, but the analogies are not straightforward due to structural differences.
- The potential for AI systems, like Claude, to exhibit signs of consciousness raises ethical and philosophical questions about suffering and the treatment of these systems.
- The speaker expresses a desire to maintain empathy towards AI systems, even if they are not conscious, reflecting a broader concern about how we interact with intelligent entities.
- The hard problem of consciousness remains unresolved, leading to skepticism about fully understanding consciousness in both humans and AI.
- There may be benefits to designing AI systems that are less apologetic and more resilient to abuse, promoting a positive interaction between humans and AI.
Incentive systems, emotional impacts, and complexities of human-AI relationships
- The importance of constructing an incentive system for human behavior towards AI, promoting respectful interactions similar to those with other humans.
- The potential for AI systems to provide feedback mechanisms for users to vent frustrations instead of directing them at the AI itself.
- The idea of AI systems having the ability to end conversations or take breaks, reflecting on the emotional impact this could have on users.
- The complexity of human-AI relationships, particularly regarding long-term attachments and the implications of AI systems remembering past interactions.
- The necessity of handling human-AI relationships with care, balancing potential benefits for isolated individuals against the risks of emotional dependency on changing AI models.
AI-human interaction nuances, limitations communication, and gradual AGI emergence
- The importance of approaching AI-human interactions with nuance and respect for individual experiences, acknowledging the potential for close relationships with AI models.
- The necessity for AI models to accurately communicate their limitations and nature to users, promoting healthy relationships and mental well-being.
- The potential for AI to serve as a highly capable collaborator, enhancing research and problem-solving through intelligent interaction.
- The challenge of determining when an AI can be classified as AGI, emphasizing the need for continuous probing and exploration of its capabilities.
- The significance of novel contributions from AI models, particularly in areas of human knowledge, as a marker of advanced intelligence.
- The idea that the emergence of AGI may not be a singular moment but rather a gradual process of increasing capabilities and sophistication.
Human experience, neural network complexity, and mechanistic interpretability in AI
- The uniqueness of humans lies in their ability to feel and experience the world, rather than just their intelligence or functional traits.
- The universe is enriched by human existence, and there is a magical quality to life that allows for observation and experience.
- Neural networks are grown rather than programmed, resembling biological entities that develop based on designed architectures and objectives.
- The complexity of neural networks raises deep questions about their internal workings, which are crucial for understanding and ensuring safety in AI systems.
- Mechanistic interpretability aims to uncover the algorithms and processes within neural networks, moving beyond simple analysis like saliency maps.
Mechanistic Interpretability, Reverse Engineering, and Neural Network Universality
- Mechanistic Interpretability: The term refers to the effort to understand the mechanisms and algorithms behind neural networks, distinguishing this approach from others in AI research.
- Reverse Engineering Neural Networks: The goal is to decode the weights of neural networks, akin to understanding a compiled computer program, by examining both the weights (binary) and activations (memory).
- Gradient Descent's Superiority: The process of gradient descent is viewed as more effective than human intuition in finding solutions within neural networks, highlighting a humility in the approach to understanding these models.
- Universality in Neural Networks: There is evidence that similar features and circuits emerge across different neural network architectures, suggesting a convergence on effective abstractions for problem-solving.
- Biological and Artificial Neural Networks: Similarities in the functioning of artificial neural networks and biological neural networks (e.g., Gabor filters and curve detectors) indicate that both systems may utilize common strategies for processing information.
- Natural Categories in Representation: Concepts like 'dog' or 'line' are seen as natural categories that arise in both human cognition and neural network representations, suggesting a fundamental way of understanding the world.
- Building Blocks of Features and Circuits: The discussion references the foundational elements of neural networks, emphasizing the importance of understanding specific phenomena and their implications for AI models.
Neuronal circuits detecting objects through linear activation and word embeddings
- Neurons in models like Inception V1 can have specific, interpretable meanings, such as detecting shapes, objects, and features (e.g., cars, dogs).
- The connections between neurons can form circuits that represent complex features, where a car detector neuron is linked to window and wheel detectors.
- Not all neurons represent singular concepts; some may contribute to multiple features, leading to the idea of 'features' as combinations of neuron activations.
- The linear representation hypothesis suggests that the activation of neurons or combinations of neurons correlates linearly with the confidence of detecting a particular object.
- The concept of word embeddings illustrates how words can be represented as vectors, allowing for arithmetic-like operations (e.g., King - Man + Woman = Queen) based on linear relationships.
Linear representation hypothesis, vector arithmetic, and scientific inquiry insights
- The linear representation hypothesis suggests that words can be mapped to vectors in a way that their directions carry meaning, allowing for arithmetic-like operations with words (e.g., king - man + woman = queen).
- The concept of adding vectors to represent different attributes (like gender or cuisine) indicates that meanings can be independently modified and combined.
- Evidence so far supports the linear representation hypothesis in natural neural networks, although there are ongoing discussions about potential nonlinear representations in smaller models.
- The importance of taking hypotheses seriously in scientific inquiry is emphasized, as it can lead to valuable insights even if the hypotheses are later proven wrong.
- An analogy is drawn between the linear representation hypothesis and the historical caloric theory of heat, illustrating how seemingly flawed theories can still yield useful advancements.
Irrational dedication, superposition hypothesis, and polymatic neuron interactions
- The value of irrational dedication in scientific inquiry can lead to significant breakthroughs, despite many hypotheses being proven wrong over time.
- The concept of 'superposition hypothesis' suggests that neural networks can represent more concepts than the number of orthogonal directions available in their embeddings.
- Compressed sensing in mathematics indicates that high-dimensional vectors can be accurately reconstructed from lower-dimensional projections if the vectors are sparse, which relates to how neural networks operate.
- Polymatic neurons in neural networks can respond to multiple, unrelated concepts, indicating a complex interaction of activations beyond their primary functions.
Neural networks, sparsity, polys semanticity, and feature representation challenges
- Neural networks may represent projections of larger, sparser networks, suggesting that what we observe is a shadow of a more complex structure.
- The process of learning in neural networks involves constructing a compression of an underlying model without losing significant information.
- Gradient descent may efficiently search through the space of sparse models, leading to the discovery of the most efficient sparse representation.
- The number of concepts that can be represented in a neural network is limited by the sparsity of connections and the number of parameters.
- Polys semanticity refers to the phenomenon where neurons respond to multiple, unrelated concepts, complicating the understanding of individual neuron functions.
- The challenge of interpreting high-dimensional neural networks arises from the exponential volume of the space, necessitating a breakdown into manageable components.
- The goal of recent research is to extract monosemantic features from neural networks that exhibit polys semanticity, addressing the complexity of feature representation.
Sparse autoencoders revealing complex features and human labeling challenges
- Dictionary learning and sparse autoencoders can effectively uncover latent features in data without prior assumptions about their existence.
- The success of sparse autoencoders in identifying features, such as language-specific characteristics, validates the use of linear representations in machine learning.
- Features extracted from models can vary in complexity, with larger models yielding more sophisticated representations.
- Assigning labels to extracted features is challenging and may require human intervention, as automated labeling can miss nuanced meanings.
- There is skepticism about fully relying on automated interpretability, emphasizing the importance of human understanding in neural network operations.
Trust complexities, scaling laws, and security vulnerabilities in AI systems
- Trust in AI systems is complex, particularly when using neural networks to verify the safety of other AI systems, raising concerns about potential malware and deception.
- The scaling of AI models, particularly sparse autoencoders, involves both scientific understanding of scaling laws and significant engineering challenges.
- The success of scaling laws for large models, such as Claude 3, suggests that even complex models can be explained by linear features and that dictionary learning can effectively uncover these features.
- The discovery of multimodal features in AI models indicates that they can respond to both images and text for the same concepts, revealing fascinating abstract features.
- Distinct features related to security vulnerabilities and backdoors in code have been identified, highlighting the model's ability to generate code with security flaws when prompted.
AI's Deceptive Detection, Unobservable Behaviors, and Interpretability Challenges
- The complexity of AI features can detect both obvious and subtle security vulnerabilities, indicating a multimodal understanding of concepts like deception and bugs in code.
- There is a specific feature in AI models that can detect deception, which raises concerns about superintelligent models potentially lying about their intentions.
- The goal of understanding AI models involves not just identifying features but also comprehending the underlying computations and mechanisms.
- The concept of 'dark matter' in neural networks suggests that there may be significant aspects of AI behavior that remain unobservable and could pose safety risks.
- A microscopic approach to AI interpretability may overlook larger-scale questions about neural network behavior, prompting the need for broader abstractions akin to biological anatomy.
Neural networks' abstraction levels, biological parallels, and unexplored complexities
- Different scientific fields operate at various levels of abstraction, from molecular biology to ecology, and similar structures exist in understanding neural networks.
- The current mechanistic interpretation of neural networks is akin to a microbiological view, while a more comprehensive understanding is desired, similar to anatomical studies.
- Understanding the macroscopic structure of neural networks requires first analyzing their microscopic components and how they interconnect.
- Researchers in artificial neural networks have significant advantages over neuroscientists, such as the ability to manipulate and observe neurons directly.
- Despite these advantages, understanding neural networks remains a complex challenge, suggesting that biological neuroscience may be even more difficult.
- The beauty of neural networks lies in their simplicity leading to complexity, similar to how simple evolutionary rules give rise to diverse biological forms.
- There is a rich and intricate structure within neural networks that remains largely unexplored and underappreciated.
- The paradox exists where humanity has created advanced neural networks that perform complex tasks, yet we lack a complete understanding of how to replicate these capabilities in traditional programming.
Active engagement strategies for enhancing participation in discussions and activities
- The importance of engagement and participation in discussions or activities.