Nvidia CEO Jensen Huang talks blowout quarter, AI, inferencing, ongoing demand, and more
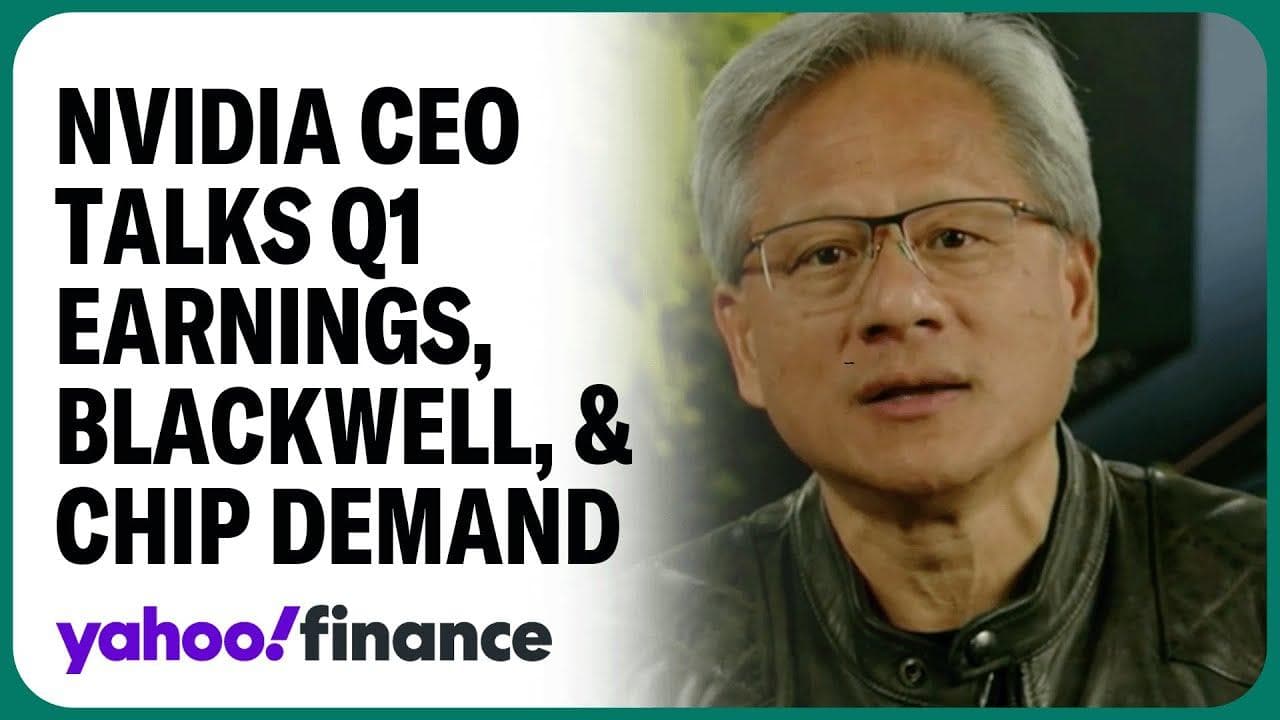
Yahoo Finance
This summary has been generated with an Alpha Preview of our AI engine. Inaccuracies may still occur. If you have any feedback, please let us know!
Interview Summary
Summary reading time: 4 minutes
☀️ Quick Takes
Is Clickbait?
Our analysis suggests that the Interview is not clickbait. It thoroughly covers Nvidia's financial performance, AI advancements, inferencing, and ongoing demand.
1-Sentence-Summary
Nvidia's CEO Jensen Huang highlights the company's robust quarter driven by the demand for their advanced AI chips like Blackwell, their dominance in the inferencing market, and the broad adoption of their technology in industries ranging from automotive to cloud computing.
Favorite Quote from the Author
the best way to teach these AIS how the physical world behaves is through video just watching tons and tons and tons of videos
💨 tl;dr
Nvidia's Q1 data center revenue skyrocketed 4,127% YoY. Announced a bullish sales forecast and a 10-for-1 stock split. Blackwell chip, designed for advanced AI, shipping this year. High demand for Hopper and Blackwell chips causing supply constraints. AI factories integrate complex systems. Major investments from Meta and Tesla. Collaborations with startups for AI applications. AI models deployed across industries. Automotive sector focuses on autonomous capabilities. Video-based training crucial for AI, especially in self-driving cars.
💡 Key Ideas
- Nvidia's first quarter data center revenue surged 4,127% year-over-year.
- Bullish sales forecast and a 10-for-1 stock split announced.
- Blackwell chip, designed for trillion parameter AI models and generative AI, shipping this year.
- Blackwell supports multiple data center configurations and ethernet, enhancing deployment.
- High demand for Hopper and Blackwell chips, causing supply constraints until next year.
- Nvidia's AI factories integrate CPUs, GPUs, memory, NVLink, Infiniband, ethernet, and complex software.
- 40% of data center revenue comes from cloud providers, with growth anticipated.
- Meta and Tesla heavily invest in Nvidia for AI and generative models.
- Collaborations with startups like Recursion for applications like drug discovery.
- AI models understand and learn various languages and domains, deployed across industries.
- Automotive is the largest vertical in Nvidia's data center business, focusing on autonomous capabilities.
- Video-based training essential for self-driving cars, requiring massive computing power.
- Similar AI tech in self-driving cars applied to large language models and physics understanding.
- Future AI will need to understand the physical world, best taught through multimodal training with video.
🎓 Lessons Learnt
- AI Spending Momentum is Strong: Nvidia's robust sales forecast and significant revenue growth indicate continued high investment in AI.
- Generative AI Needs Advanced Chips: The complexity of generative AI requires high-performance, adaptable chips like Nvidia's Blackwell.
- Inferencing is a Major Market: The complex nature of AI inferencing presents a significant market opportunity for Nvidia.
- Supply Constraints Reflect Demand: High demand for Nvidia's Hopper and Blackwell chips is causing supply constraints, showing strong market interest.
- AI Factories are Holistic Units: Nvidia's integrated AI systems, which combine CPUs, GPUs, and advanced networking, are highly complex and in demand.
- Versatile Chip Architecture is Essential: Nvidia's adaptable architecture supports continuous innovation, crucial for handling growing AI model demands.
- Major Companies Heavily Invest in AI: Companies like Meta and Tesla are investing significantly in Nvidia technology, highlighting its critical role in AI advancements.
- Revolutionary Applications of Generative AI: Generative AI is transforming sectors like autonomous driving and drug discovery.
- Autonomous Features are Future of Automotive: Future cars will require autonomous capabilities for various functions.
- Video Data is Key for AI Training: Training AI with video is much more effective than using labeled images, necessitating high computing power.
- AI Must Understand the Physical World: Next-gen AI models need to be grounded in real-world data, with video being the most effective medium for this training.
🌚 Conclusion
Nvidia is leading the AI revolution with massive revenue growth and advanced chip technology. High demand and supply constraints highlight market interest. Major companies and startups are heavily investing in Nvidia's AI solutions. Autonomous driving and generative AI are key focus areas, with video data playing a crucial role in training AI models. Nvidia's integrated AI systems and versatile chip architecture are essential for future AI advancements.
Want to get your own summary?
In-Depth
Worried about missing something? This section includes all the Key Ideas and Lessons Learnt from the Interview. We've ensured nothing is skipped or missed.
All Key Ideas
Nvidia's Recent Developments and Announcements
- Nvidia's fiscal first quarter saw data center revenue soar by 4,127% year-over-year, and the company gave a bullish sales forecast.
- Nvidia announced a 10-for-1 forward stock split and raised its dividend.
- Blackwell, Nvidia's next-generation chip, is shipping this year and expected to generate significant revenue.
- Blackwell is designed for trillion parameter AI models and generative AI, requiring high performance for tasks like prediction of tokens, pixels, and frames.
- Blackwell supports various data center configurations, including air-cooled, liquid-cooled, x86, and the new Grace processor.
- Blackwell's versatility includes support for ethernet data centers, expanding deployment possibilities beyond the Hopper generation.
- Generative AI has significantly increased the complexity of inferencing, making Nvidia's architecture crucial for ongoing innovation and competitiveness.
Nvidia's Market and Technology Insights
- Inference is a complicated problem with a giant market opportunity for Nvidia
- Vast majority of inferencing today is done on Nvidia
- Nvidia will be supply constrained for Hopper and Blackwell chips until next year due to high demand
- Nvidia builds AI factories, not just GPU chips
- AI factories consist of CPUs, GPUs, memory, NVLink, Infiniband, ethernet switches, and complex software
- AI factories are built as a holistic unit but can be disaggregated for various data center architectures
- 40% of Nvidia's data center revenue comes from cloud providers
- Both cloud providers and other industries are expected to grow in their use of Nvidia technology
- Meta and Tesla are heavily investing in Nvidia technology for AI and generative models
- Nvidia collaborates with startups like Recursion for advanced applications such as drug discovery
AI and Autonomous Technology Insights
- AI models can understand and learn almost any language, including English, images, video, chemicals, protein, and physics
- This AI capability is being deployed at scale in various industries
- Automotive is now the largest vertical within Nvidia's data center business
- Every car will eventually need autonomous capability for safety and convenience
- Learning from video directly is the most effective way to train self-driving car models
- Training from video requires enormous computing power due to high data rates
- Similar AI technology used in self-driving cars is applied to grounding large language models in understanding physics
- Future AI needs to understand the physical world, best taught through video
- This multimodality training will drive significant computing demand in the coming years
All Lessons Learnt
Key Points on AI and Chip Technology
- AI Spending Momentum is Strong: The continued bullish sales forecast and significant revenue growth in Nvidia's data center segment indicate that AI spending is robust and growing.
- Generative AI is Complex: Generative AI, which involves prediction and generation of content, has made inferencing significantly more complicated. This requires advanced and high-performance chips.
- Adaptability of Blackwell Chips: The new Blackwell chips are designed to be highly adaptable, fitting into various data center setups and supporting both traditional and new types of data centers like ethernet data centers.
- Need for Advanced Processing: As AI models grow in size and complexity, with parameters doubling every six months, there's a critical need for high-performance processing to keep up with these advancements.
- Versatility in Chip Architecture is Key: Nvidia's versatile architecture allows for continuous innovation in AI, making it possible to handle the increasing demands of generative AI.
Key Insights on Nvidia's Market and Technology
- Inferencing is a major market opportunity: Inference is complex, with complicated software stacks and various models, making it a significant market for Nvidia.
- Supply constraints due to high demand: Nvidia is facing supply constraints for Hopper and Blackwell chips due to overwhelming demand, indicating strong market interest.
- AI factories as holistic units: Nvidia builds AI factories as integrated systems with CPUs, GPUs, complex memory, and intricate networking, which are then disaggregated for different data centers.
- High complexity of AI factory components: The components of Nvidia's AI factories are among the most complex computers ever made, leading to supply constraints.
- Growth in both cloud providers and other industries: Both cloud providers and various other industries are expected to grow as they adopt Nvidia chips for generative AI and large language models.
- Significant investments by major companies: Companies like Meta and Tesla are heavily investing in Nvidia technology for large-scale AI projects, indicating the tech's critical role in future advancements.
- Generative AI's revolutionary applications: Generative AI is being used in groundbreaking ways, such as Tesla's end-to-end self-driving and Recursion's drug discovery, showcasing its transformative potential.
Key Insights on AI and Automotive Industry
- Automotive Industry Requires Autonomous Capability: Every car in the future will need autonomous features for safety, convenience, and enjoyment.
- Training AI with Video is Highly Effective: Using video data directly to train AI models is much more effective than using labeled images.
- AI Needs to Understand Physical World: Next-gen AI must be grounded in the physical world, and video is the best medium to teach this.
- High Computing Demand for AI Training: The need for multimodal AI training, especially with video, will significantly increase computing demands in the future.